Houman Owhadi
CalTech
Seminar Information
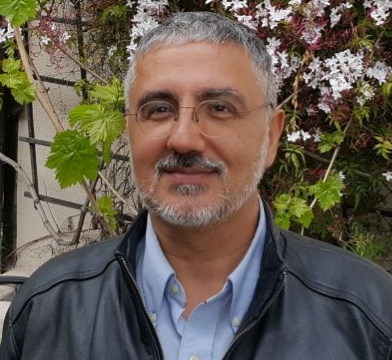
We introduce a framework for generating, organizing, and reasoning with computational knowledge. It is motivated by the observation that most problems in Computational Sciences and Engineering (CSE) can be described as that of completing (from data) a computational graph representing dependencies between functions and variables. Functions and variables may be known, unknown, or random. Data comes in the form of observations of distinct values of a finite number of subsets of the variables of the graph (satisfying its functional dependencies). The underlying problem combines a regression problem (approximating unknown functions) with a matrix completion problem (recovering unobserved variables in the data). Replacing unknown functions by Gaussian Processes (GPs) and conditioning on observed data provides a simple but efficient approach to completing such graphs. Since the proposed framework is highly expressive, it has a vast potential application scope. Since the completion process can be automatized, as one solves $\sqrt{\sqrt{2}+\sqrt{3}}$ on a pocket calculator without thinking about it, one could, with the proposed framework, solve a complex CSE problem by drawing a diagram. Compared to traditional regression/kriging, the proposed framework can be used to recover unknown functions with much scarcer data by exploiting interdependencies between multiple functions and variables. We illustrate the scope and efficacy of the proposed framework by showing how it can be used as a pathway to identifying simple solutions to classical CSE problems.
Prof. Houman is a professor of applied and computational mathematics and control and dynamical systems at the California Institute of Technology. His interests include uncertainty quantification, numerical approximation, statistical inference/learning, data assimilation, stochastic and multiscale analysis. His current research has been focused (since 2013) on solving numerical approximation problems as learning problems, learning problems as numerical approximation problems, and uncertainty quantification problems as adversarial games. He was a plenary speaker at SIAM CSE 2015, a tutorial speaker at SIAM UQ 2016 and the recipient of the 2019 Germund Dahlquist Prize (SIAM). His research is supported by DARPA, the Department of Energy, NASA/JPL, AFOSR, ONR, National Nuclear Security Administration, Los Alamos National Laboratory, Beyond Limits, and the National Science Foundation.