Alyssa Kody
Argonne National Laboratory
Seminar Information
Seminar Recording Available: Please contact seminar coordinator, Jake Blair at (j1blair@eng.ucsd.edu)
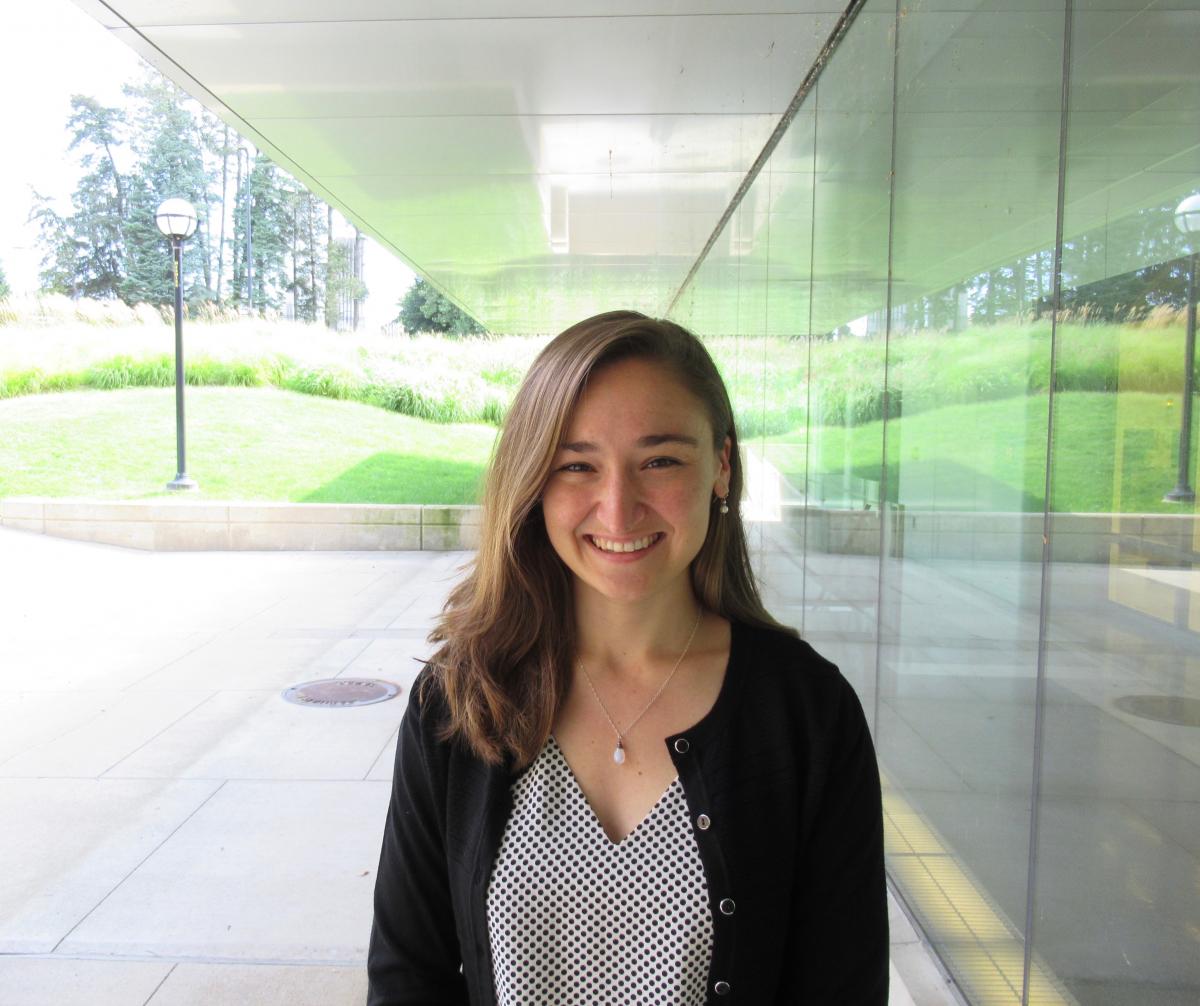
To operate a reliable, resilient, and cost-effective electric grid, operators and planners will need to quickly and accurately solve challenging optimization and control problems. To provide reliable power to customers over the coming decades, system operators must contend with increased complexity (e.g., nonlinearities, mixed-integer models) in operational and planning algorithms. Furthermore, the combination of an aging electric infrastructure and the growing threat of extreme weather events makes resilience considerations increasingly imperative. Accordingly, this talk has two focuses: (1) the specialized and targeted use of machine learning to increase the accuracy and computation speeds of power systems decision-making algorithms, and (2) the development of analysis and computational tools to enhance energy infrastructure resilience to extreme weather events. The first half of this talk with focus on works related to machine learning-enhanced optimization for power systems, where I target the use of machine learning to aid in solving nonlinear optimization problems. The second half of the talk will focus on wildfire risk mitigation for power systems through scheduled de-energizations during high-wildfire-risk periods. I will conclude the talk by discussing ongoing work using machine learning methods for wildfire risk mitigation in power systems.
Alyssa Kody is a Maria Goeppert Mayer Postdoctoral Fellow at Argonne National Laboratory in Lemont, Illinois. Her research focuses on developing control and optimization algorithms for power and energy systems. She received her Ph.D. in Electrical Engineering from the University of Michigan in Ann Arbor in 2019, where her thesis was on developing control systems for self-powered technologies. Her graduate work was supported by a National Science Foundation Graduate Research Fellowship and a Rackham Merit Fellowship.