Ching-Yao Lai
Stanford University
Seminar Information
Engineering Building Unit 2 (EBU2)
Room 479
Seminar Recording Available: Please contact seminar coordinator, Jake Blair at (j1blair@ucsd.edu)
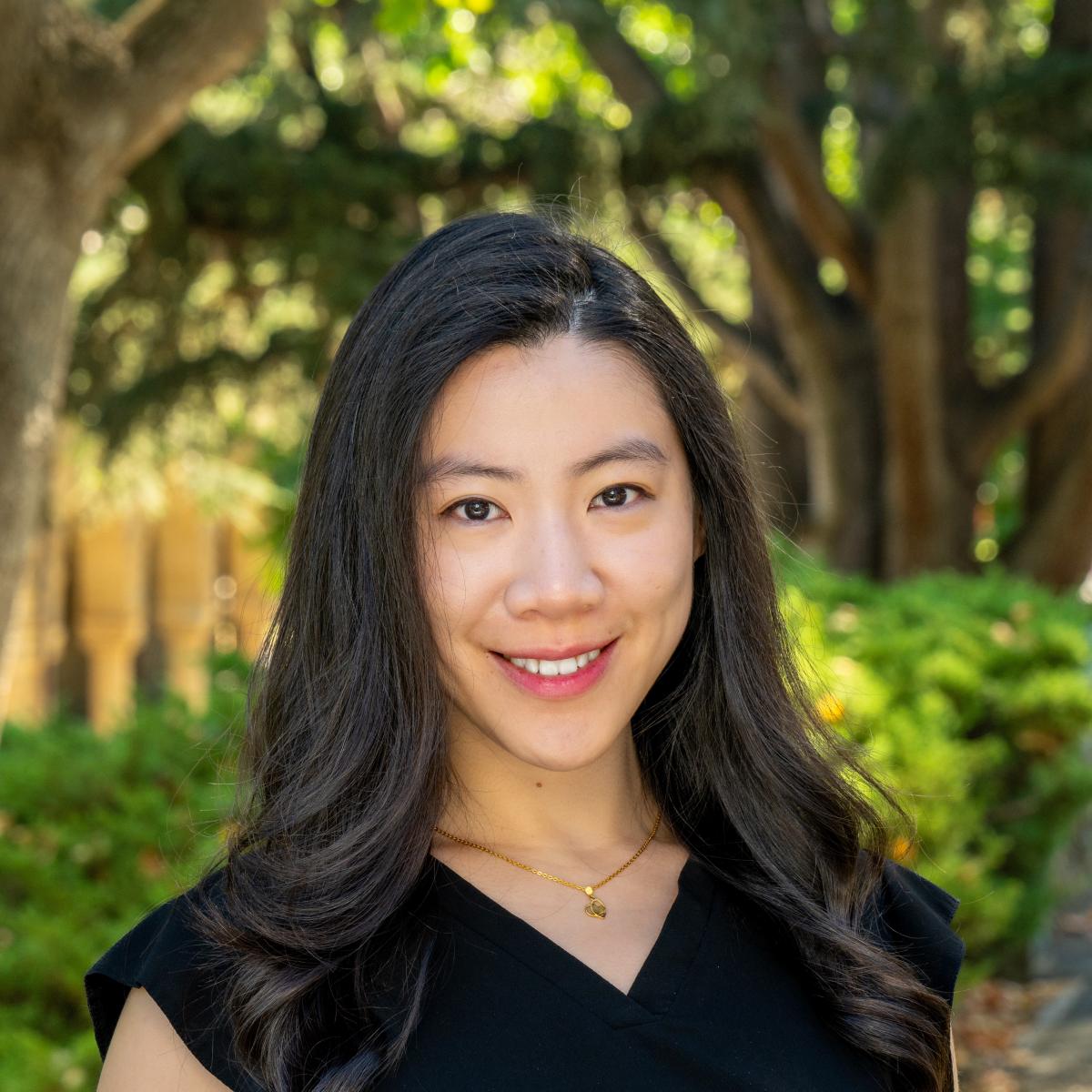
Deep learning techniques are increasingly being applied to scientific problems where network precision is crucial. Despite being considered universal function approximators, neural networks often struggle with optimization issues in practice. We developed an algorithm to tackle this issue and achieve machine-precision representation (Wang and Lai, JCP 2024). I will discuss two examples in fluid dynamics using neural networks (NN) to approximate partial differential equations (PDEs) solutions and solve inverse problems. The first example concerns the search for singularities of the Euler equations (Wang-Lai-Gómez-Serrano-
Ching-Yao Lai (Yao) is an Assistant Professor in the Department of Geophysics and an Affiliated Faculty of the Institute for Computational and Mathematical Engineering (ICME) at Stanford. Before joining Stanford, she was an Assistant Professor at Princeton University. She received an undergraduate degree (2013) in Physics from National Taiwan University and a PhD (2018) in Mechanical and Aerospace Engineering from Princeton University. She completed her postdoctoral research in geophysics at Lamont Earth Observatory at Columbia University where she received the Lamont Postdoctoral Fellowship. Her current research focuses on enhancing the representation of machine-learning models to tackle multiscale problems. She was the recipient of the 2023 Google Research Scholar Award and the 2024 Sloan Research Fellowship.